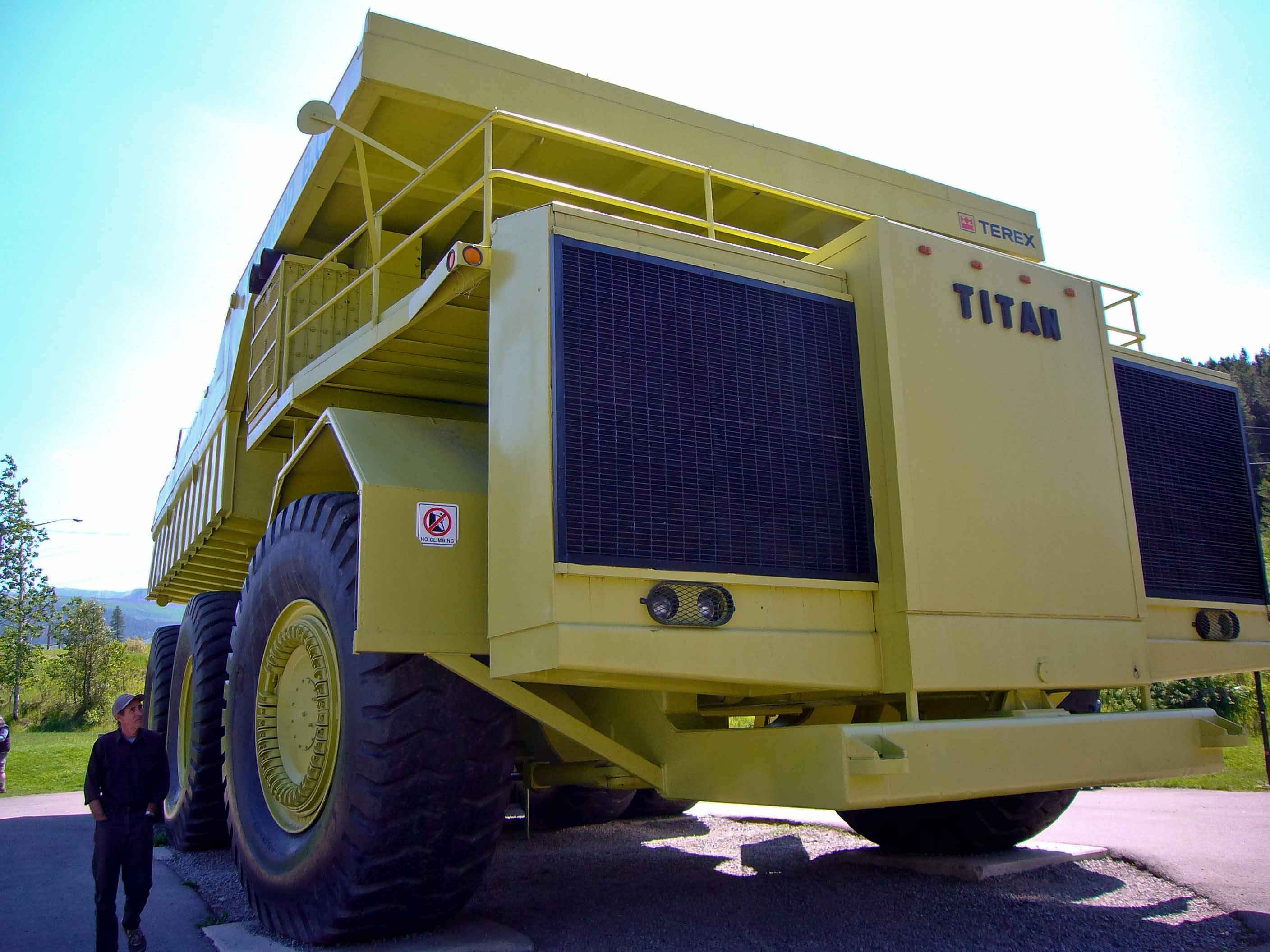
Photo Credit: sashafatcat via Compfight cc
He hated going to the gardening store. Though his vehicle was capable of carrying the supplies, such things as gravel, sand, and flagstones, it was such a hassle to maneuver it. On one trip, a moment of inattention resulted in damage down the entire side of a minivan in the next parking spot over. One other time, he backed too deeply into the spot, and the protruding vehicle took out the picture window in the front of the store. He apologized profusely, and paid for the window. Yet patrons and the owner only glowered at him, pointing at his vehicle and exclaiming, “Really? REALLY?”
He knew it was more than he needed, but he might need that capacity later, so he bought the biggest one available. He knew it might be difficult to run and handle, but he bought the very best. Why were people so judgmental about it? And of course he was keenly aware of the cost of operating it. Friends had approached him, suggesting that something like a Ford F150 would be much more appropriate, and affordable, even if he had to make multiple trips.
He supposed they were correct, but he hated to admit it. And he hated the thought of selling the best, big vehicle. Settling for something so mainstream and boring as a pickup truck just turned his stomach. Especially when he so enjoyed the pride of ownership of a vehicle with a Caterpillar or TEREX name plate.
Our little story above may seem far fetched. Who would drive a giant construction haul truck to the local gardening store? One would hope no one would do that. But in many ways, the “Big Data” craze is driving people to overdrive their data, using a construction haul truck instead of something that might be more suitable, even if it is larger than the pickup truck in our story.
The importance of choosing the right sized solution is even more important in Information Security, since it is an overhead expense that reduces a firm’s bottom line. Vendors are pushing “Big Data” as the solution to every analytic problem. Some security problems are well served by these tools, but they are expensive and complex. A small Hadoop cluster typically has 20 to 50 nodes. Storage, network, nodes, memory, power, cooling, software, administrators… these costs will ramp up very quickly. Even if the cluster is “in the Cloud” the virtual images take real storage, real power, and a real slice of physical hardware. Too often the initial requirement of a “Big Data” project is to have all logs in original format available for unlimited analysis. This will bloat the implementation size significantly, but often isn’t the real requirement, which is greater and more flexible visibility into operations as evidenced by computer logs.
Many firms have found that effective and efficient analysis on logs can be done on a “Medium Data” basis that provides actionable results at far lower cost. Indeed, such analysis should be standard practice to determine if larger platforms are really needed, and to ensure that the larger systems are correctly sized and tasked.
Don’t succumb to the “Big Data” craze. Prove out the need for larger systems with smaller samples and more traditional techniques. If you need the larger tools, you will know it. And if you don’t need the big tools, you won’t waste valuable resources and be left in the dust by more nimble competitors.